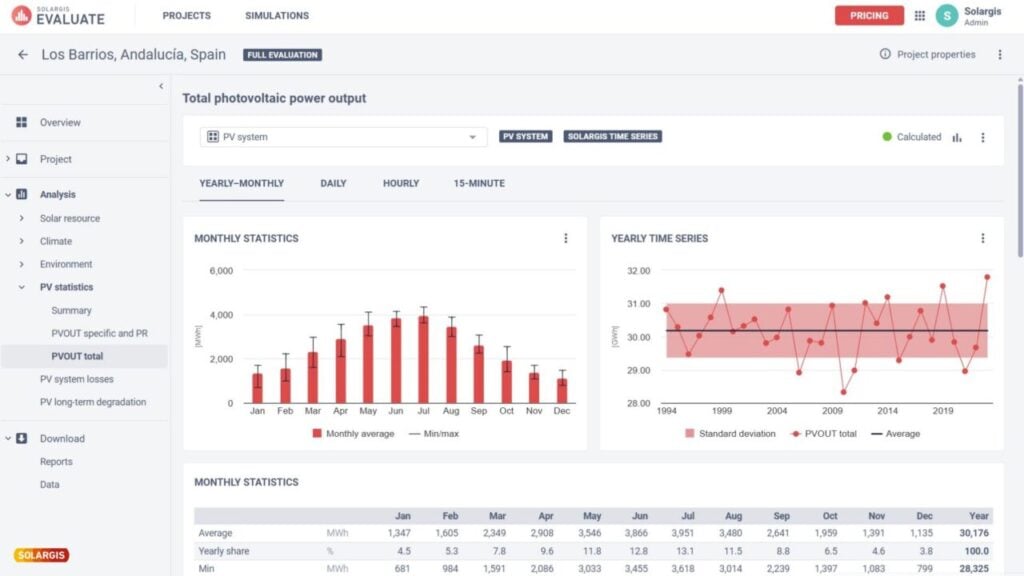
For years, the solar industry has relied on Typical Meteorological Year (TMY) data as the standard for PV simulations and energy yield predictions. But as solar projects become more complex and extreme weather events become more frequent, it is clear that TMY’s limitations are holding the industry back.
Just as we once moved from standard-definition television to 4K resolution, it’s time to upgrade the way we model performance of PV systems. In the solar market, accuracy is alpha and omega to secure financing, optimise PV plant design, reduce risks and ensure reliable performance. This demands more detailed evaluation than TMY is able to provide.
Unlock unlimited access for 12 whole months of distinctive global analysis
Photovoltaics International is now included.
- Regular insight and analysis of the industry’s biggest developments
- In-depth interviews with the industry’s leading figures
- Unlimited digital access to the PV Tech Power journal catalogue
- Unlimited digital access to the Photovoltaics International journal catalogue
- Access to more than 1,000 technical papers
- Discounts on Solar Media’s portfolio of events, in-person and virtual
Or continue reading this article for free
Unlike TMY, time series provides higher granularity of data, and a more realistic picture of the solar and meteorological conditions. This transition is critical for improving return on investment (ROI) from photovoltaics and overall project bankability. It is time to accept time series data as the new standard.
Skewing energy yield predictions
TMY datasets aggregate historical data into a ’typical’ year, virtually averaging out variability. While TMY is still relevant in certain use cases, such as. in the pre-feasibility phase of PV development, it is much less accurate in optimising power plant performance.
In recent years, we are witnessing extreme weather events becoming more frequent. Increasing occurrences of heatwaves, wildfires, storms and periods with lower irradiance can significantly impact solar energy production.
In September 2024, for example, Portugal experienced massive forest fires, reducing Global Horizontal Irradiance (GHI) by up to 30% due to thick smoke, followed by increased PV module soiling. Events like these show us why a single ’typical’ year cannot accurately represent the full range of conditions that PV plants must endure.
In cases like these, we can actively observe that relying on low-granularity data can skew predictions. The practice of using hourly granularity of TMY fails to capture short-term irradiance fluctuations, leading to overly optimistic energy yield estimates.
Capturing short-term and long-term variability
The key advantage of time series data is its ability to account for both short-term (intra-hourly) and long-term (interannual) variability, providing a more accurate foundation for PV performance modelling.
Short-term variability, such asrapid fluctuations in solar radiation that occur within minutes, is caused by passing clouds, fast changing weather patterns, or storms. These variations cannot be captured in the hourly TMY datasets.
By using 15-minute time series data, developers can better model inverter behavior and design systems resilient to sudden condition changes. This ensures that grid operators and developers can anticipate real-time performance variations, reducing the risk of voltage fluctuations and instability in PV plant operations.
When it comes to long term, or interannual, variability, such as natural atmospheric and hydrological cycles, it causes significant differences in solar radiation year over year. While TMY averages out these changes, time series data allows developers to compare each year individually at a more granular level.
For PV developers, this variability directly affects revenue expectations. Financial models that rely on TMY averages fail to anticipate potential revenue fluctuations, putting investors at risk. By contrast, Time Series data allows for detailed year-on-year comparisons, providing a more robust basis for financial modelling and risk assessment.
High-resolution data as a key to enhancing project bankability
One of the most critical aspects of PV project development is securing funding from banks and investors. Projects that rely on simplified TMY data models often fail to gain the confidence of lenders about long-term performance estimates.
Investors and financiers are becoming increasingly cautious about weather-related risks. With extreme weather events and climate variability becoming more evident, relying on TMY-based PV simulations introduces unnecessary risk. Time series data, on the other hand, provides a full picture of expected revenue fluctuations, including worst-case and best-case scenarios, and better alignment with investor expectations, ensuring that PV projects are evaluated with realistic performance estimates.
It is important to note that, like any other industry, the solar industry is and should be evolving. It’s not wise for us to rest on our laurels and stick to the outdated practices and legacy approaches.
Using the right datasets for the right tasks is essential—TMY for pre-feasibility work and time series for accurate project evaluation. Both developers and investors require greater accuracy to optimise PV performance. Adopting high-resolution time series data at this project phase is not just an improvement—it’s the necessary industry shift.